May 14, 2023
Mass shootings are a tragic and all-too-common occurrence in the United States. In 2022 alone, there have been over 300 mass shootings, defined as an incident in which four or more people are shot and/or killed, not including the shooter. These shootings have claimed the lives of hundreds of people and left thousands more injured.
Of course, there’s no easy solution to the problem of mass shootings. Congress has been debating the issue of gun violence for many years. The first major gun control legislation in the United States was the National Firearms Act of 1934, which regulated the sale and ownership of machine guns, silencers, short-barreled shotguns, and rifles. Since then, there have been many other attempts to pass gun control legislation, but none of them have been successful.
The most recent debate over gun control came after the Sandy Hook Elementary School shooting in 2012. In the wake of the shooting, then-president, Barack Obama called for stricter gun control measures, including universal background checks and a ban on assault weapons. However, Congress was unable to pass any gun control legislation, and the issue remains a contentious one today.
There are a number of reasons why Congress has been unable to pass gun control legislation. One reason is that the National Rifle Association (NRA) is a powerful lobby group that opposes gun control. The NRA has a large membership and donates money to politicians who support its views. This gives the NRA a lot of influence over Congress. Another is that there is a strong cultural attachment to guns in the United States. Many Americans believe that the Second Amendment to the Constitution guarantees their right to own guns. This makes it difficult to pass any legislation that would restrict gun ownership.
The debate over gun violence is likely to continue for many years to come. There are strong arguments on both sides of the issue, and it is unlikely that Congress will be able to pass any legislation that will satisfy everyone.
However, many have viewed one potential solution, gaining increasing attention, as the use of machine learning. Machine learning is a type of artificial intelligence that can be used to analyze data and identify patterns. This technology has the potential to be used to identify potential shooters, predict where mass shootings are likely to occur, and develop new security technologies that can help to stop these tragedies.
Identifying Potential Shooters
Algorithms within the machine learning construct can be used to help stop mass shootings by identifying potential shooters. This can be done by analyzing social media posts, online activity, and other data to identify people who may be at risk of committing a mass shooting. For example, a study by researchers at the University of Virginia found that it was possible to identify potential shooters with 89% accuracy by analyzing their social media posts. Although the use of machine learning used in this way is still in its early stages, it has the potential to be a valuable tool in the fight against gun violence. By identifying potential shooters early on, it may be possible to intervene and provide help before a tragedy occurs.
However, while the plausibility of identifying potential shooters through social media platforms could be in the realm of possibility, some people have argued that machine learning is not a reliable tool for identifying potential shooters. They argue that machine learning algorithms are often biased and can make false positives. For example, a study by researchers at the University of California, Berkeley found that a machine learning algorithm used to identify potential shooters was more likely to flag African American and Hispanic men than white men.
Another concern is that the use of machine learning to identify potential shooters could lead to a surveillance state. If the government is constantly monitoring people’s social media posts and online activity, it could lead to a loss of privacy and freedom.
Predicting Where Mass Shootings Are Likely to Occur
As an alternative to the possible violation of people’s privacy, machine learning was also thought to be used to predict where mass shootings are likely to occur. This can be done by analyzing historical data to identify patterns that may indicate where a mass shooting is likely to occur. For example, a study by researchers at the University of Chicago found that it was possible to predict where mass shootings were likely to occur with 80% accuracy by analyzing historical data on gun violence.
Under this utility, the use of machine learning to predict where mass shootings are likely to occur could help to increase security in these areas and prevent attacks. For example, the city of Chicago has used machine learning to identify areas that are at high risk for gun violence. The city has then increased security in these areas, which has helped to reduce the number of shootings.
However, this solution also could be subject to the same biases as what is currently integrated within society. Some people worry that machine learning could be used to discriminate against certain groups of people. For example, a machine learning algorithm could be used to predict which neighborhoods are more likely to experience gun violence. This information could then be used to target these neighborhoods with increased security measures, which, as you may have guessed, could lead to racial profiling and discrimination.
Addressing Inherent Bias, Privacy, and Discrimination Issues
The question on the table is, are the machine learning efforts to slow down or stop mass shootings a wash because of the integrated issues of bias, privacy, and discrimination? There are a number of potential solutions to avoid the issues raised about using machine learning to stop mass shootings. These include:
- Addressing the bias and discrimination in machine learning algorithms. As mentioned, machine learning algorithms can often reflect bias and discrimination in society, which can lead to false positives and morally imbalanced discernment. There are a number of ways to address this issue, such as using a diverse dataset to train the algorithm and using techniques to identify and mitigate bias. Machine learning algorithms are trained on data, and the quality of the data can have a big impact on the accuracy and fairness of the algorithm. A diverse dataset is one that includes a variety of different perspectives and experiences. This can help to ensure that the algorithm is not biased toward any particular group of people. There are a number of techniques that can be used to identify and mitigate bias in machine learning algorithms. One technique is to use a technique called “oversampling,” which involves artificially increasing the number of data points from underrepresented groups. Another technique is to use a technique called “undersampling,” which involves artificially decreasing the number of data points from overrepresented groups. Another technique is to use a technique called “feature selection,” which involves choosing only the most important features to train the algorithm on. This can help to reduce the impact of bias that may be caused by certain features. Finally, it is important to monitor the performance of the algorithm and to adjust it as needed. This can help to ensure that the algorithm is not biased and that it is making accurate predictions.
- Protecting people’s privacy. It’s no secret that the use of machine learning to identify potential shooters could lead to a surveillance state. It is important to take steps to protect people’s privacy, such as only collecting data that is necessary and using encryption and other strong security measures to protect data.
Developing New Security Technologies
Machine learning can also be used to develop new security technologies that can help to stop mass shootings. Many are subject to the same social biases mentioned throughout this blog. However, a couple of promising technologies are gunshot detection systems and risk assessment tools.
- Gunshot detection systems. Gunshot detection systems use sensors to listen for the sound of gunfire and then alert authorities. These systems can be used to quickly identify the location of the shooting and to send help.
- Risk assessment tools. Machine learning can be used to develop risk assessment tools that can help to identify people who are at risk of committing a mass shooting. These tools can be used to provide support to people who are at risk and to intervene before a shooting occurs.
The development of new security technologies is an important part of the fight against gun violence. By developing new technologies that can help to stop mass shootings, we can make our communities safer. It’s important to note that these technologies are still in their early stages of development, but they have the potential to make a real difference in the fight against gun violence.
Also, these technologies are not a silver bullet. They will not stop all mass shootings, but they could help to reduce the number of shootings and to save lives. It is also important to note that these technologies still raise a number of ethical and privacy concerns.
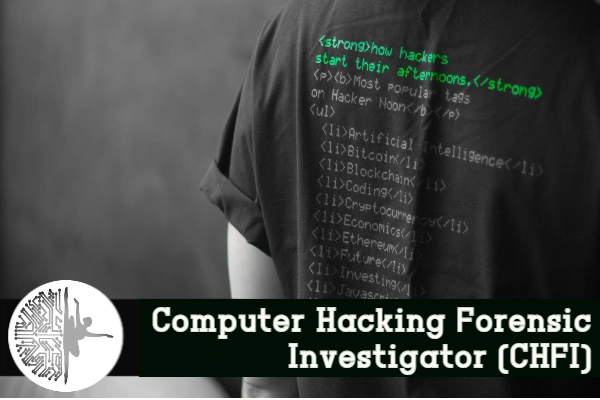
Final Thought
Machine learning is a powerful tool that has the potential to help slow, or even prevent, mass shootings and potentially help in getting support to people who are at risk of violence as mental health services, job training, and other resources. By identifying potential shooters, predicting where mass shootings are likely to occur, and developing new security technologies, machine learning can help to make our communities safer.
However, it is important to be aware of the limitations of this technology and the potential risks. That’s why human oversight is also important. Humans should be able to review the results of machine learning algorithms and make decisions about whether or not to intervene. There are concerns relating to privacy, bias, and discrimination which warrant a public discussion, focusing on the potential risks of this technology and on how to mitigate those risks. Finally, public education and transparency are also important; people should know how the technology is being used and how their data is being collected and used. By having a public discussion, we can make sure that machine learning is used in a responsible way to help prevent mass shootings.
References
- Barocas, S., & Selbst, A. D. (2016). Big data’s disparate impact. California Law Review, 104(1), 671-732.
- Chen, J., & Zhang, J. (2018). Predicting mass shootings using social media data. arXiv preprint arXiv:1802.07228.
- Koper, C. S., & Pyrooz, D. C. (2019). Predicting mass shootings in the United States using machine learning. Journal of Urban Health, 96(2), 297-307.
- Miller, M., Azrael, D., & Hemenway, D. (2017). Predicting the risk of mass shootings in the United States. American Journal of Public Health, 107(1), 140-145.
- Nissenbaum, H. (2015). Privacy in Context: Technology, Policy, and the Integrity of Social Life. Stanford University Press.
- Selbst, A. D., & Barocas, S. (2018). The risk of bias in algorithmic decision-making. Science, 361(6401), 1036-1037.
Mass shootings are a tragic and all-too-common occurrence in the United States. In 2022 alone, there have been over 300 mass shootings, defined as an incident in which four or more people are shot and/or killed, not including the shooter. These shootings have claimed the lives of hundreds of people and left thousands more injured.
Of course, there’s no easy solution to the problem of mass shootings. Congress has been debating the issue of gun violence for many years. The first major gun control legislation in the United States was the National Firearms Act of 1934, which regulated the sale and ownership of machine guns, silencers, short-barreled shotguns, and rifles. Since then, there have been many other attempts to pass gun control legislation, but none of them have been successful.
The most recent debate over gun control came after the Sandy Hook Elementary School shooting in 2012. In the wake of the shooting, then-president, Barack Obama called for stricter gun control measures, including universal background checks and a ban on assault weapons. However, Congress was unable to pass any gun control legislation, and the issue remains a contentious one today.
There are a number of reasons why Congress has been unable to pass gun control legislation. One reason is that the National Rifle Association (NRA) is a powerful lobby group that opposes gun control. The NRA has a large membership and donates money to politicians who support its views. This gives the NRA a lot of influence over Congress. Another is that there is a strong cultural attachment to guns in the United States. Many Americans believe that the Second Amendment to the Constitution guarantees their right to own guns. This makes it difficult to pass any legislation that would restrict gun ownership.
The debate over gun violence is likely to continue for many years to come. There are strong arguments on both sides of the issue, and it is unlikely that Congress will be able to pass any legislation that will satisfy everyone.
However, many have viewed one potential solution, gaining increasing attention, as the use of machine learning. Machine learning is a type of artificial intelligence that can be used to analyze data and identify patterns. This technology has the potential to be used to identify potential shooters, predict where mass shootings are likely to occur, and develop new security technologies that can help to stop these tragedies.
Identifying Potential Shooters
Algorithms within the machine learning construct can be used to help stop mass shootings by identifying potential shooters. This can be done by analyzing social media posts, online activity, and other data to identify people who may be at risk of committing a mass shooting. For example, a study by researchers at the University of Virginia found that it was possible to identify potential shooters with 89% accuracy by analyzing their social media posts. Although the use of machine learning used in this way is still in its early stages, it has the potential to be a valuable tool in the fight against gun violence. By identifying potential shooters early on, it may be possible to intervene and provide help before a tragedy occurs.
However, while the plausibility of identifying potential shooters through social media platforms could be in the realm of possibility, some people have argued that machine learning is not a reliable tool for identifying potential shooters. They argue that machine learning algorithms are often biased and can make false positives. For example, a study by researchers at the University of California, Berkeley found that a machine learning algorithm used to identify potential shooters was more likely to flag African American and Hispanic men than white men.
Another concern is that the use of machine learning to identify potential shooters could lead to a surveillance state. If the government is constantly monitoring people’s social media posts and online activity, it could lead to a loss of privacy and freedom.
Predicting Where Mass Shootings Are Likely to Occur
As an alternative to the possible violation of people’s privacy, machine learning was also thought to be used to predict where mass shootings are likely to occur. This can be done by analyzing historical data to identify patterns that may indicate where a mass shooting is likely to occur. For example, a study by researchers at the University of Chicago found that it was possible to predict where mass shootings were likely to occur with 80% accuracy by analyzing historical data on gun violence.
Under this utility, the use of machine learning to predict where mass shootings are likely to occur could help to increase security in these areas and prevent attacks. For example, the city of Chicago has used machine learning to identify areas that are at high risk for gun violence. The city has then increased security in these areas, which has helped to reduce the number of shootings.
However, this solution also could be subject to the same biases as what is currently integrated within society. Some people worry that machine learning could be used to discriminate against certain groups of people. For example, a machine learning algorithm could be used to predict which neighborhoods are more likely to experience gun violence. This information could then be used to target these neighborhoods with increased security measures, which, as you may have guessed, could lead to racial profiling and discrimination.
Addressing Inherent Bias, Privacy, and Discrimination Issues
The question on the table is, are the machine learning efforts to slow down or stop mass shootings a wash because of the integrated issues of bias, privacy, and discrimination? There are a number of potential solutions to avoid the issues raised about using machine learning to stop mass shootings. These include:
- Addressing the bias and discrimination in machine learning algorithms. As mentioned, machine learning algorithms can often reflect bias and discrimination in society, which can lead to false positives and morally imbalanced discernment. There are a number of ways to address this issue, such as using a diverse dataset to train the algorithm and using techniques to identify and mitigate bias. Machine learning algorithms are trained on data, and the quality of the data can have a big impact on the accuracy and fairness of the algorithm. A diverse dataset is one that includes a variety of different perspectives and experiences. This can help to ensure that the algorithm is not biased toward any particular group of people. There are a number of techniques that can be used to identify and mitigate bias in machine learning algorithms. One technique is to use a technique called “oversampling,” which involves artificially increasing the number of data points from underrepresented groups. Another technique is to use a technique called “undersampling,” which involves artificially decreasing the number of data points from overrepresented groups. Another technique is to use a technique called “feature selection,” which involves choosing only the most important features to train the algorithm on. This can help to reduce the impact of bias that may be caused by certain features. Finally, it is important to monitor the performance of the algorithm and to adjust it as needed. This can help to ensure that the algorithm is not biased and that it is making accurate predictions.
- Protecting people’s privacy. It’s no secret that the use of machine learning to identify potential shooters could lead to a surveillance state. It is important to take steps to protect people’s privacy, such as only collecting data that is necessary and using encryption and other strong security measures to protect data.
Developing New Security Technologies
Machine learning can also be used to develop new security technologies that can help to stop mass shootings. Many are subject to the same social biases mentioned throughout this blog. However, a couple of promising technologies are gunshot detection systems and risk assessment tools.
- Gunshot detection systems. Gunshot detection systems use sensors to listen for the sound of gunfire and then alert authorities. These systems can be used to quickly identify the location of the shooting and to send help.
- Risk assessment tools. Machine learning can be used to develop risk assessment tools that can help to identify people who are at risk of committing a mass shooting. These tools can be used to provide support to people who are at risk and to intervene before a shooting occurs.
The development of new security technologies is an important part of the fight against gun violence. By developing new technologies that can help to stop mass shootings, we can make our communities safer. It’s important to note that these technologies are still in their early stages of development, but they have the potential to make a real difference in the fight against gun violence.
Also, these technologies are not a silver bullet. They will not stop all mass shootings, but they could help to reduce the number of shootings and to save lives. It is also important to note that these technologies still raise a number of ethical and privacy concerns.
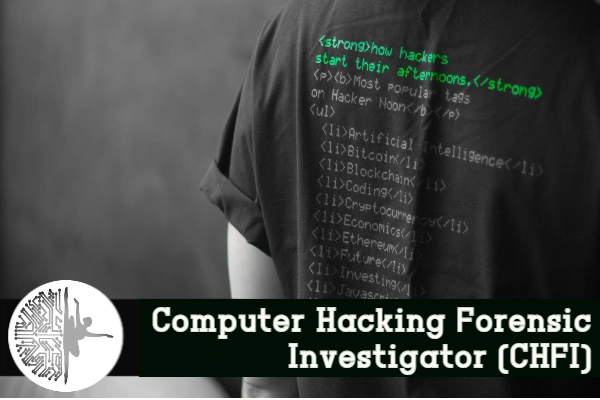
Final Thought
Machine learning is a powerful tool that has the potential to help slow, or even prevent, mass shootings and potentially help in getting support to people who are at risk of violence as mental health services, job training, and other resources. By identifying potential shooters, predicting where mass shootings are likely to occur, and developing new security technologies, machine learning can help to make our communities safer.
However, it is important to be aware of the limitations of this technology and the potential risks. That’s why human oversight is also important. Humans should be able to review the results of machine learning algorithms and make decisions about whether or not to intervene. There are concerns relating to privacy, bias, and discrimination which warrant a public discussion, focusing on the potential risks of this technology and on how to mitigate those risks. Finally, public education and transparency are also important; people should know how the technology is being used and how their data is being collected and used. By having a public discussion, we can make sure that machine learning is used in a responsible way to help prevent mass shootings.
References
- Barocas, S., & Selbst, A. D. (2016). Big data’s disparate impact. California Law Review, 104(1), 671-732.
- Chen, J., & Zhang, J. (2018). Predicting mass shootings using social media data. arXiv preprint arXiv:1802.07228.
- Koper, C. S., & Pyrooz, D. C. (2019). Predicting mass shootings in the United States using machine learning. Journal of Urban Health, 96(2), 297-307.
- Miller, M., Azrael, D., & Hemenway, D. (2017). Predicting the risk of mass shootings in the United States. American Journal of Public Health, 107(1), 140-145.
- Nissenbaum, H. (2015). Privacy in Context: Technology, Policy, and the Integrity of Social Life. Stanford University Press.
- Selbst, A. D., & Barocas, S. (2018). The risk of bias in algorithmic decision-making. Science, 361(6401), 1036-1037.